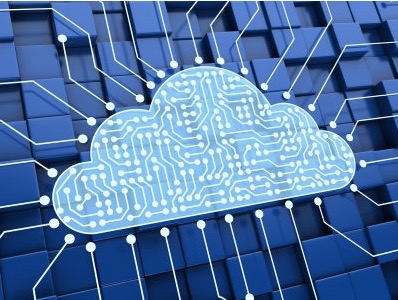
In my childhood, I was taught that truth triumphs. My grandfather always used to say that there is only one version of truth. Lies may be told many different ways, but there is no chance of having a second version of true fact. I believed this to be true, until I grew older.
Today, I wonder if there can be different versions of the same fact from different parties. Is one party lying about the fact? Sometimes it seems that way. But to be fair to everyone, more often than not, people, even politicians, do not lie about a fact. In today’s ever connected world of cellphones and tablets, they would be caught immediately. People can verify the truth with modern technology. It is literally at their fingertips! Typically, people, especially politicians present the same fact but from a different perspective so the outcome is perceived differently by the audience. Perception is technically not a lie. So, mastering the techniques to create a desired perception is a desired trait, particularly for politicians!
I know that many of us have strong opinions regarding politicians. But sometimes I wonder if we are guilty of similar behavior. We all use techniques to achieve a favorable outcome, without the risk of being called an outright liar. We use these techniques everyday with clients, coworkers and even family members.
Modern era of business is driven by data. Data is considered the best asset of a company. It is considered the real estate or intellectual property of a business. Companies are spending hundreds of millions of dollars in infrastructure, data and application architecture to enable data driven business decisions so the errors of decisions on gut feel are avoided.
Analytics are built on the visual representation of underlying data to support decision making in real time (or near real time). But visual representation can be tricky. Analytics visualization can misrepresent the facts unless they are built with the business purpose in mind. A lot of manipulation can be done to the visual presentation that in the end misrepresents the underlying fact. Sales metrics used to present year over year sales data can be manipulated easily to show sales growth when there isn’t any, simply by manipulating the time period or scale of the graph. Sometimes, improper analytics can help to suppress a problem or highlight a potential non-issue thereby dragging entire focus towards it.
Design of analytics for presenting business parameters and metrics should take as much priority as identification of the metrics. But most organizations spend significantly more effort on defining the metrics, latency, underlying data availability and completeness than they spend on the analytics itself. Often technical teams end up designing metrics with little or no input from business users to ensure that they can be built in the analytic tool. But this can be problematic as technical teams may not have a full understanding of the use of the analytics on the decision making process. Business users should have strong participation in designing the analytics, even if the technical team is responsible for designing them.
With the line between the business and technical team responsibility diminishing in the data & analytics space, business users are nowadays more involved in defining the metrics and analytics. Organizations are focused more on self-service analytics platform, where the technical team is responsible for creating the infrastructure for analytics, ensuring that data is delivered at proper grain in a timely fashion on platform with business users responsible for analytics building and delivery. This helps to improve the utilization and effectiveness of analytics for business users. Design of analytics is better owned by business users. Ultimately, analytics helps drive business decisions. Technical teams can support analytics by solving technical challenges with infrastructure and data.
By: Kajal Mukhopadhyaya, Principal Solutions Architect